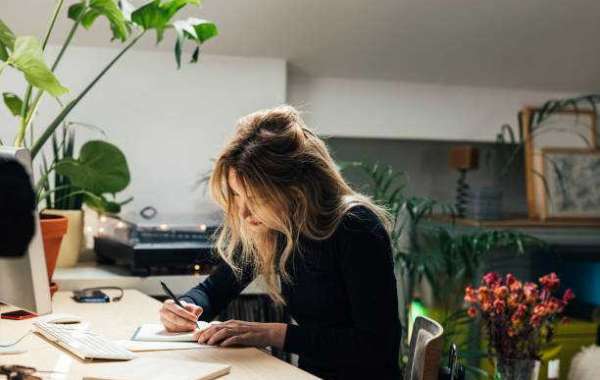
Data Collection and Analysis in Ph.D. Research: Best Practices
Ph.D. research is a significant undertaking that demands rigorous planning, execution, and analysis.
At the heart of any Ph.D. project lies the collection and analysis of data, a process that can make or break the quality and credibility of the research.
In this era of big data and advanced analytical tools, the importance of best practices in data collection and analysis cannot be overstated.
This article delves into the essential best practices that PhD dissertation researchers should adhere to for robust data collection and analysis, ensuring their research stands on a solid foundation.
Defining Clear Research Objectives
The journey of data collection and analysis in Ph.D. research begins with a well-defined research question or hypothesis.
Without a clear understanding of what you aim to investigate or prove, collecting relevant data becomes challenging.
Take the time to refine your research objectives, ensuring they are specific, measurable, achievable, relevant, and time-bound (SMART). This clarity will guide your data collection efforts and help you stay on track throughout the research process.
Choosing the Right Data Sources
Selecting appropriate data sources is essential to your research’s success. Depending on your research question, data can be sourced from various places, including surveys, interviews, experiments, existing datasets, and archival records.
The choice of data sources should align with your research objectives and methodology. It’s essential to evaluate the reliability and validity of your chosen data sources to ensure the accuracy of your findings.
Developing a Robust Data Collection Plan
A well-structured data collection plan is the cornerstone of effective data gathering. This plan should outline the following:
- Data collection methods: Specify the techniques and tools you’ll use to collect data. This might include surveys, interviews, observations, or experiments.
- Data collection instruments: Detail the instruments and questionnaires you’ll employ, ensuring they are designed to capture the necessary information accurately.
- Sampling strategy: Define your target population, the sampling method (random, stratified, purposive, etc.), and the sample size. Justify your choices based on the research objectives.
- Data collection timeline: Create a schedule that outlines when and how data will be collected. This helps in managing resources efficiently.
Ensuring Data Quality and Integrity
Maintaining data quality and integrity is paramount. By implementing the following measures, you can safeguard your quantitative data:
Data validation: Cross-check data for errors, inconsistencies, and missing values during and after collection.
Data security: Protect sensitive and confidential information by employing encryption and access controls.
Backup procedures: Regularly back up your data to prevent data loss due to technical failures or human errors.
Ethical Considerations in Data Collection
Ethical considerations play a crucial role in data collection. Ensure that your research complies with ethical standards and regulations, including obtaining informed consent from participants, ensuring privacy and confidentiality, and mitigating any potential harm to participants. Seek approval from your institution’s ethics review board if required.
Data Pre-processing
Raw data often requires pre-processing to make it suitable for analysis. Common pre-processing steps include:
Data cleaning: Identifying and rectifying errors, outliers, and missing values.
Data transformation: Converting raw data into a suitable format for analysis, such as scaling or normalizing variables.
Data integration: Combining data from various sources if necessary.
Data Analysis Methodology
Selecting the appropriate data analysis methods is crucial. This decision should be guided by your research objectives and the nature of your data. Common data analysis techniques include:
Descriptive statistics: Summarizing and visualizing data to understand its basic characteristics.
Inferential statistics: Drawing conclusions about populations based on sample data, including hypothesis testing and confidence intervals.
Qualitative analysis: Analyzing non-numeric data, such as text, to identify patterns and themes.
Advanced analytical techniques: Employing complex methods like regression analysis, factor analysis, or machine learning when necessary.
Maintaining Transparency and Reproducibility
To ensure the credibility of your research, maintain transparency and reproducibility throughout the data analysis process.
Document your methods, assumptions, and decision-making processes. Provide clear explanations of your analyses in your research reports to enable others to replicate your findings. Utilize open-source software and share your code whenever possible.
Data Visualization
Effective data visualization is key to conveying your findings in a comprehensible and persuasive manner. Use graphs, charts, and tables to present your data visually and ensure that they are clear, well-labelled, and directly related to your research questions. Avoid visual clutter and misleading representations.
Iterative Analysis
Data analysis is seldom a linear process. Be prepared to iterate and revisit your analysis as you uncover new insights or face challenges. Seek feedback from peers, advisors, or mentors to refine your analysis and ensure its robustness.
Interpretation and Discussion
Once you have analyzed your data, it’s time to interpret the final results and discuss their implications. Provide context for your findings and relate them back to your research objectives. Address any limitations in your data or methodology and consider alternative explanations for your results.
Drawing Conclusions and Recommendations
Based on your analysis and interpretation, draw well-founded conclusions that directly address your research question or hypothesis. Offer practical recommendations for future research or real-world applications if applicable.
Peer Review and Feedback
Before finalizing your research, seek peer review and feedback from experts in your field. Constructive criticism can help identify weaknesses in your data analysis and interpretation, ensuring the quality of your work.
Data Storage and Archiving
Ensure that your data is properly archived and stored in a secure and accessible manner. This not only facilitates transparency and reproducibility but also ensures the longevity of your research.
Continuous Learning
The field of data collection and analysis is continually evolving. Stay updated on the latest methodologies, software tools, and best practices to enhance the quality of your research.
Conclusion
In conclusion, the meticulous process of data collection and analysis serves as the backbone of Ph.D. research, and its adherence to best practices is paramount for producing research that is both credible and influential. From establishing precise research objectives and selecting appropriate data sources to formulating a robust data collection plan and upholding ethical standards, these foundational steps pave the way for reliable results.
Please sign in below
Did you forget your password? Click here
Don't have an account yet? Register here